Live
- 525 candidates remain in fray for 17 LS seats in Telangana
- Political Prowess on Display: Rajnath Singh and Smriti Irani's nominations galvanise BJP’s poll campaign
- TN government committee identifies 42 elephant corridors in state
- Ola Cabs CEO Hemant Bakshi steps down, firm announces job cuts
- IPL 2024: Kuldeep Yadav's 35 not out propels Delhi to 153/9 against Kolkata
- BJP leader Sambit Patra files nomination for Puri Lok Sabha seat
- Rajiv Pratap Rudy accuses Lalu Prasad of 'killing' developmental schemes in Saran
- Quick action by Telangana Cyber Security Bureau saves citizen's Rs 1 crore
- Types of food diabetics should avoid
- Celebrating National Honesty Day: 20 Quotes to Inspire Integrity
Just In
How generative AI can help cyber security teams tackle the skills gap
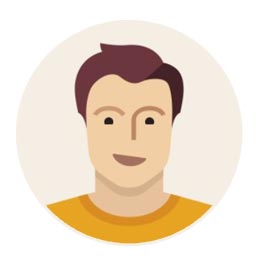
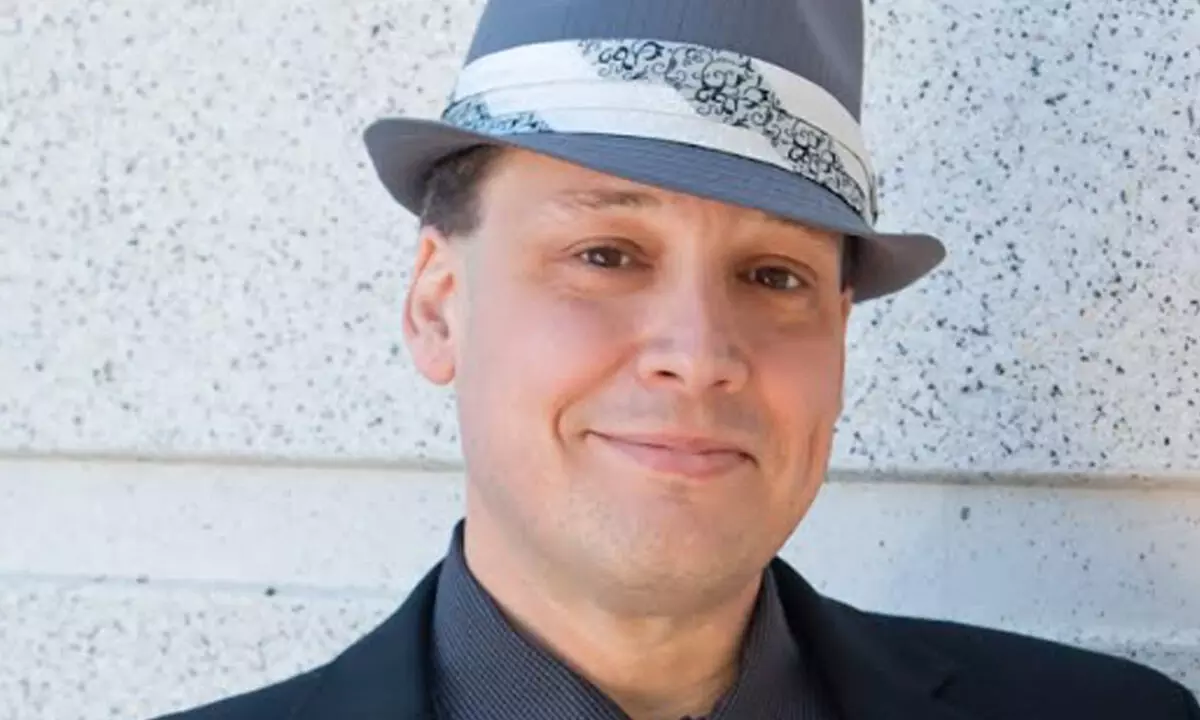
Cybersecurity challenges are escalating on a global scale, compounding the difficulties faced by already strained and overburdened cybersecurity teams.
Cybersecurity challenges are escalating on a global scale, compounding the difficulties faced by already strained and overburdened cybersecurity teams. The industry's pervasive skills gap further exacerbates these challenges, presenting a major issue for businesses not only in India but worldwide. Four in ten organisations in India grapple with understaffed cybersecurity teams and the predicament goes beyond mere staffing concerns. A staggering 68% of organisations in the country encounter difficulties in retaining talent within cybersecurity teams.
Despite organisations increasing their cybersecurity budgets and implementing upskilling initiatives, the current skills gap remains vast, making it more difficult to effectively address the surging number of cybersecurity threats. In this landscape, Generative AI has emerged as a potent solution to bridge this gap. Here's how.
Why organisations need Generative AI for cybersecurity
Constrained by limited resources, existing cybersecurity teams often find themselves in a reactive stance, responding to cyberattacks rather than proactively preventing them. A commissioned study conducted by Forrester Consulting on behalf of Tenable reveals that 73% of respondents believe their organisations would achieve greater success in defending against cyberattacks if they could allocate more resources to preventive cybersecurity measures.
The adoption of generative AI-powered cybersecurity platforms serves as a powerful force multiplier, allowing for a faster way to analyse large numbers of assets, vulnerabilities, threats and other datasets to quickly identify where risks exist within the organisation and provide context that is otherwise difficult to take into account. Consider the difference between looking at an asset inventory and identifying 100 laptops in the environment that all have vulnerabilities that need to be patched. Including more data and context can help teams prioritise where they should deploy patches first, but manually taking additional data and context into account is time-consuming and leaves the organisation open to attack while trying to decide where to start. Generative AI tools can take in many other large data sets of information about the assets which can quickly provide crucial context for an analyst, moving an analysis of a group of 100 laptops to identifying the two laptops that belong to the CEO and CFO, contain administrative access, store critical business data and are allowed to access key backend databases of customer data. Bringing that level of content and relationship immediately to a security professional’s view means they can more quickly decide where to focus and prioritise patching these key systems to mitigate the most amount of overall business risk.
These kinds of automated and contextualised results also help address the skills gap by allowing more junior analysts and security professionals to easily identify and understand the business-specific context of a threat or finding. It encourages organisations to adopt a preventive approach to cybersecurity, by making those junior team members more effective quickly and allows senior members to be more efficient and effective at applying their historical knowledge of the environment to identify more hard-to-find threats and security issues. Ultimately, when applied judiciously, generative AI can empower cybersecurity teams by improving their efficiency and reallocating resources toward more strategic tasks, particularly in the realm of thwarting potential attacks.
Of course, the efficacy of generative AI is contingent upon the quality of the underlying datasets. For this technology to function as an effective cybersecurity ally, it must possess the capability to accurately identify patterns and automate crucial actions, thereby making preventive cybersecurity a scalable proposition. If implemented incorrectly, generative AI may have adverse effects on organisations, as any inaccuracies in pattern recognition can lead to a mere semblance of security rather than actual protection.
How to choose the right generative AI solution for a cybersecurity assistant?
Generative AI assistants rely heavily on contextual data for their effectiveness. When seeking cybersecurity solutions featuring generative AI integration, opting for technologies built on extensive datasets that provide a deep contextual understanding of assets, vulnerabilities, business impact and more is crucial. This ensures that preventive security recommendations, whether for on-premises environments, cloud infrastructure, or public-facing assets, are not only effective but also accurate.
Deprived of contextual data, generative AI becomes incapable of aiding resource-constrained security teams in preventing successful attacks and providing swift analysis and guidance. Choosing the right platform becomes a significant advantage for organisations committed to establishing a robust preventive cybersecurity program.
Generative AI as a force multiplier
Generative AI can also serve as a valuable tool for senior security practitioners, streamlining the analysis of extensive data sets to extract essential information. It simplifies the analysis process by offering a comprehensive evaluation of assets and exposures within environments, understanding the contextual risks, and prioritising remediation efforts. And, if a Large Language Model (LLM) interface is available on the front-end of the analysis engine, practitioners can simply pose natural language search queries to obtain relevant insights saving time and preventing the need to learn specific query languages or platform-specific context to search through the datasets.
Cybersecurity teams are tasked with evaluating multiple factors such as exposure specifics, asset characteristics, user privileges, external accessibility, and attack paths contribute to determining the priority of fixing vulnerabilities and misconfigurations. Generative AI significantly eases this process, reducing the time required for analysing exposures across all of the different findings. For example, when investigating exposure to Log4Shell, a cybersecurity team can effortlessly inquire, "How many assets have Log4j installed?" or "How many assets with Domain Administrator access have Log4j installed?", and the GenAI engine can correlate and create the context across all the datasets to answer the question quickly and easily.
Generative AI conducts detailed analyses quickly, providing concise summaries of exposure and risk. This empowers security teams to make better decisions about how to mitigate these risks by offering insights into high-risk users, vulnerable systems, and likely attack paths, allowing them to prioritise remediation efforts and more quickly execute whatever mitigation method is decided upon.
The applications of generative AI in cybersecurity extend beyond analysis, encompassing capabilities such as debugging, log parsing, anomaly detection, triaging, and incident response. It proves beneficial for reverse engineers, aids development teams in static code analysis, and identifies potentially exploitable code. With advanced threat detection and intelligence from trained AI models, developers can identify broken code more quickly and earlier in the development process allowing them to fix the code long before it ever reaches QA, testing or production.
This means that organisations can implement a more effective preventative security program into their development and DevOps teams. This proactive approach enables cybersecurity teams to identify and address issues preemptively, giving defenders more time to address the problem, and thus, giving attackers fewer attack vectors for cybercriminals to attempt breaching their networks. Despite the existing skills gap, leveraging generative AI emerges as a potent solution to enhance cybersecurity defences.
(The author is Chief Security Strategist, Tenable)

© 2024 Hyderabad Media House Limited/The Hans India. All rights reserved. Powered by hocalwire.com