Live
- Casual yet stylish office outfits for all-day comfort
- TTD to suspend all special darshans from January 10 to 19 amid Vaikuntha dwara darshans
- Naidu pats TDP leaders, cadres for enrolling 73L members
- Rupali Ganguly says for 20 years she never got an award
- Advanced anti-drone systems deployed for devotees’ safety at Mahakumbh
- Workshop on ‘Industry-Academia Practices in Civil Engineering’ concludes
- Revanth assures Kurma community of its due
- 204 cadets pass out of AFA
- Youngest chess king wins laurels for India
- FairPoint: Rahul’s rhetoric falls flat as PM Modi steals spotlight
Just In
Revolutionizing Insurance with AI and Behavioural Analytics: An Interview with Vinod K. Singh, Co-founder & CTO of Concirrus
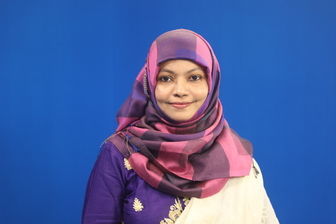

Concirrus, under the leadership of Co-founder and CTO Vinod K. Singh, has addressed this gap with its AI-powered platform, Quest. Quest enables insurers to move beyond outdated models by leveraging real-time behavioural data for more precise risk assessments.
The insurance industry has traditionally relied on static data models based on demographic factors and historical information, such as age or location. These models often fail to assess risk because they overlook dynamic behavioural data—how an asset is used or the real-time conditions it faces accurately. As a result, many insurers struggle with inefficiencies in policy pricing and risk management. Additionally, the sheer volume of data generated today has become a challenge for insurers, as they often lack the technology to process and analyze it effectively. Although AI and machine learning could offer valuable insights, the cost and complexity of implementing these advanced technologies have slowed their adoption in the sector.
Concirrus, under the leadership of Co-founder and CTO Vinod K. Singh, has addressed this gap with its AI-powered platform, Quest. Quest enables insurers to move beyond outdated models by leveraging real-time behavioural data for more precise risk assessments. It ingests and analyzes vast datasets, such as asset usage patterns and environmental factors, providing insights that allow for more accurate underwriting. The platform’s use of AI and machine learning streamlines the processing of large data volumes, making it both efficient and cost-effective. By adopting Quest, insurers can enhance policy pricing accuracy and improve operational efficiency.
Vinod K Singh, Co-Founder & CTO of Concirrus
How do you see AI and behavioural analysis reshaping the future of industries like insurance? What are the most significant changes you anticipate and how might this change affect how businesses operate?
I strongly believe that AI and behavioural analysis are set to bring transformative changes to the insurance industry, with the most significant shifts expected in pricing, operational efficiency, claims handling, and customer service. AI will enable insurers to develop highly personalized and dynamic pricing models, where premiums adjust in real-time based on an individual’s behavior, such as driving patterns or lifestyle choices. This move towards precision pricing will not only improve risk assessment but also enhance customer satisfaction by aligning premiums more closely with actual risk. The ability to tailor policies to specific behaviours will likely lead to increased customer loyalty and retention.
Operational efficiency across the insurance value chain will also see substantial improvements with AI. AI can significantly improve operational efficiency during the underwriting process, particularly in the pre-bind and quote-to-bind stages. By automating data collection and analysis, AI can rapidly assess risk factors from diverse sources, reducing the time required to evaluate applications. AI-driven tools can instantly analyze applicant information, compare it against historical data, and generate risk profiles with high accuracy, allowing underwriters to focus on more complex cases. Claims handling, traditionally slow and manual, will become faster and more accurate through AI-driven automation, reducing processing times, operational costs, and human error. Enhanced fraud detection is another key benefit, as AI can identify complex patterns of fraudulent behavior that might be missed by human analysts. On the distribution side, AI will enable more targeted marketing and sales strategies by analyzing customer behavior and preferences, allowing insurers to reach the right customers with the right products. These changes will fundamentally alter how businesses operate, pushing them to invest heavily in AI and data analytics capabilities, embrace a more customer-centric approach, and focus on transparency and data security to maintain consumer trust.
What role do you believe DevOps will play in enabling companies to scale AI and ML solutions effectively? How critical is this integration for the future of tech?
I think DevOps will play a pivotal role in enabling companies to scale AI and ML solutions effectively. By integrating DevOps practices with AI/ML workflows, organizations can automate and streamline the deployment, monitoring, and management of these solutions, ensuring they are reliable, scalable, and continuously improving. DevOps facilitates the continuous integration and continuous delivery (CI/CD) of AI models, allowing for rapid experimentation, testing, and iteration. This is crucial for AI/ML, where models need to be regularly updated with new data and retrained to maintain accuracy.
The integration of DevOps with AI/ML is critical for the future of tech because it bridges the gap between data science and production environments, ensuring that AI solutions are not just developed in isolation but are effectively operationalized at scale. This integration also enhances collaboration between development, operations, and data science teams, leading to more efficient workflows and faster time-to-market for AI-driven innovations. As AI becomes increasingly central to business strategies, the ability to scale these solutions efficiently will be a key differentiator, making DevOps an essential component of future technological advancements.
What challenges do you foresee as more companies adopt AI in their DevOps practices, and how can they overcome these hurdles?
It's hard to pinpoint a few as there will be technical as well as cultural challenges in my view, DevOps and MLOps have traditionally operated in silos, focusing on non-AI and AI aspects of technology, respectively. While this division was understandable in the early days of AI, it's becoming increasingly clear that as AI-driven solutions become central to tech, a more unified approach is needed. DevOps and MLOps are converging to create a comprehensive framework for teams working with AI.
As more companies integrate AI into their DevOps practices, they may encounter several challenges. First, the complexity of AI models can lead to a lack of transparency, making it difficult for teams to understand how decisions are made within their systems. This opacity can create trust issues and hinder effective troubleshooting when problems arise. To overcome this, companies should prioritize explainable AI (XAI) techniques, ensuring that their AI-driven processes are interpretable and understandable by Data Scientists, developers, and operations teams.
Another challenge is the potential for AI models to perpetuate or even amplify biases present in the data they're trained on. In a DevOps context, this could lead to unfair or suboptimal decision-making, particularly in areas like automated incident response or resource allocation. Companies can address this by implementing robust data governance practices, regularly auditing their models for bias, and incorporating diverse datasets that reflect a wide range of scenarios.
Finally, integrating AI into DevOps may require significant changes to existing workflows and skillsets, which can be met with resistance from teams accustomed to traditional methods. Overcoming this hurdle involves investing in continuous education and training for DevOps teams, fostering a culture of collaboration between AI specialists and DevOps practitioners, and gradually introducing AI tools in a way that complements, rather than disrupts, established processes. By addressing these challenges proactively, companies can harness the full potential of AI in their DevOps practices.
What impact do you think the growing demand for AI and ML expertise will have on the tech workforce, and how can companies address the talent gap?
Historically, much of software development focused on digitizing existing processes. Documents were scanned, data was entered into databases, and user interfaces were created to provide access. The shift towards AI marks a new era. Software is no longer just a tool; it's becoming the worker. From automating routine tasks to generating creative content, AI is capable of performing a wide range of functions that were once the exclusive domain of human workers.
This transformation has significant implications for the labour market. While there is concern about job displacement, AI is also creating new opportunities. As software becomes more sophisticated, there will be a growing demand for skilled professionals who can develop, maintain, and deploy AI systems. Moreover, AI can help to augment human capabilities, enabling workers to focus on more complex and strategic tasks.
“The future labour market will likely consist of new "AI jobs," alongside traditional roles, reflecting a blend of human and machine collaboration”. As more industries seek to integrate AI and machine learning into their operations, the demand for skilled professionals in these fields will far outpace supply. This talent gap can lead to intense competition for qualified individuals, driving up salaries and making it harder for companies, especially startups and smaller firms, to attract and retain top talent.
Companies can adopt several strategies to address the talent gap. First, investing in upskilling and reskilling existing employees can help bridge the gap by developing in-house talent. Offering continuous learning opportunities, such as workshops, courses, and certifications in AI and ML, can empower current staff to transition into these roles.
How can industries balance the drive for innovation with the need to maintain ethical standards and regulatory compliance in AI deployment?
Balancing innovation with ethical standards and regulatory compliance in AI involves integrating clear ethical guidelines into the development process, focusing on fairness, transparency, accountability, and privacy. Establishing a robust governance framework with cross-functional teams—comprising AI experts, ethicists, and legal advisors—ensures that ethical and regulatory aspects are managed throughout the AI lifecycle.
Adopting explainable AI practices is crucial for transparency, helping stakeholders understand decision-making processes, and supporting compliance with regulations. Continuous monitoring and auditing of AI systems are essential for detecting and addressing biases or privacy issues, ensuring ongoing alignment with ethical standards.
Incorporating a "human-in-the-loop" approach further enhances ethical oversight by involving human judgment in critical decision points, helping to address concerns that AI alone might miss. This approach, combined with fostering a culture of responsibility and engaging with regulators, enables companies to innovate responsibly while upholding ethical and regulatory standards.
What emerging trends in AI do you believe will have the most profound impact on industries in the coming years?
There are several emerging AI trends that hold strong potential to have a profound impact on industries in the coming years, but my top 3 picks are
● Generative AI stands out for its potential to transform content creation, from generating text and media to designing new molecules for drug discovery. It also enables highly personalized experiences by tailoring products and services to individual preferences, which can significantly enhance customer engagement and satisfaction.
● AI-powered automation is another key trend. Robotic Process Automation (RPA) streamlines repetitive tasks, improving efficiency, while Intelligent Process Automation (IPA) integrates AI with RPA to handle more complex processes. Autonomous vehicles are set to revolutionize transportation, logistics, and manufacturing trust in AI systems by making their decision-making processes understandable and fair. This trend is crucial for fostering ethical AI practices and ensuring compliance with regulatory standards.
● Edge AI is advancing real-time data processing by enabling data to be processed locally, which speeds up responses and enhances privacy by reducing data transmission and storage risks, and we see more and more AI on the devices we use on a regular basis, such as our phones.
Given your experience, what do you think are the key qualities needed to lead tech innovation successfully in today’s rapidly changing landscape?
I think leading tech innovation successfully in today’s rapidly changing landscape requires a blend of essential qualities and strategic approaches. First and foremost, a visionary leader must have a clear understanding of future technological trends and how they align with long-term strategic goals. However, it’s crucial to stay grounded and avoid getting swept up in the hype surrounding new technologies. Leaders should be able to differentiate between the real capabilities of technology and its limitations, ensuring that they are not overestimating its potential.
● A successful leader must also possess adaptability to navigate the fast-paced tech environment. This involves being open to new technologies and adjusting touring by enhancing efficiency and safety.
● Explainable AI (XAI) addresses the need for transparency strategies based on evolving market demands. Technical expertise remains fundamental, as it enables leaders to make informed decisions and guide their teams effectively. Yet, understanding the practical application of technology is equally important—identifying specific challenges first, and then evaluating how technology can address these challenges is essential.
It’s vital to assess the return on investment (ROI) of any technology considered. Even if a technology has the potential to solve a problem, the costs associated with developing and implementing the solution must be weighed against the benefits it brings. Leaders need to ensure that the value derived from a technological solution justifies the investment and that the benefits outweigh the costs
What advice would you give to tech professionals looking to make a meaningful impact in the AI and ML space?
I think it’s crucial to stay updated with the latest advancements while grounding your work in solid computer science fundamentals. The field of AI and ML evolves rapidly, so continuous learning and skill enhancement are essential to remain relevant and effective. Deepening your understanding of core concepts, algorithms, and techniques will not only improve your ability to apply current technologies but also ease the adoption of new ones as they emerge.
It’s important to focus on solving real-world problems rather than getting caught up in the hype surrounding new technologies. By identifying specific challenges and applying AI and ML solutions to these problems, you ensure that your work is practical and impactful. Additionally, exercise your own judgment to evaluate the potential impact of technological developments on your business or career, rather than simply following trends. Balancing theoretical knowledge with practical experience, maintaining ethical considerations, and measuring the real-world impact of your work will help you make a significant and lasting contribution to the field.

© 2024 Hyderabad Media House Limited/The Hans India. All rights reserved. Powered by hocalwire.com